AI - ML - Bioinformatics
We are interested in understanding pathogenic bacterial evolution at various scales.
We track the emergence and transmission of pathogens using Bayesian phylodynamic approaches informing improved infection control and highlighting threads to human health.
We uncover bacterial niches and transmission routes through deriving distinct mutational spectra. We then aim to de-convolute mutation spectra into the underlying mutational signatures using statistical decomposition methods and experimentally characterize the contribution of the innate DNA-repair pathways and exposure of bacteria to mutagens.
We derive molecular signatures of host adaptation using statistical genetics within and across patients and work on understanding the nature of pathogenic evolution using structural genomics and saturation mutagenesis.
We are interested in deriving a mechanistic understanding of host adaptation by functional characterisation of the key drivers of pathoadaptation, integration of genomic, transcriptomic and phenotypic data employing GWAS, multi-omics integration and causal learning methods relying on multi-scale characterisation of bacterial transposon and knock-out libraries as well as clinical isolate collections. We reconstitute evolutionary trajectories in isogenic controls as observed in human isolates.
We use machine learning to model bacterial sequence composition to predict clinically relevant phenotypes, such as AMR.
Collaborators
Julian Parkhill
Roger Levesque
Nicholas Thomson
Josie Bryant
Lucas Boeck
Publications
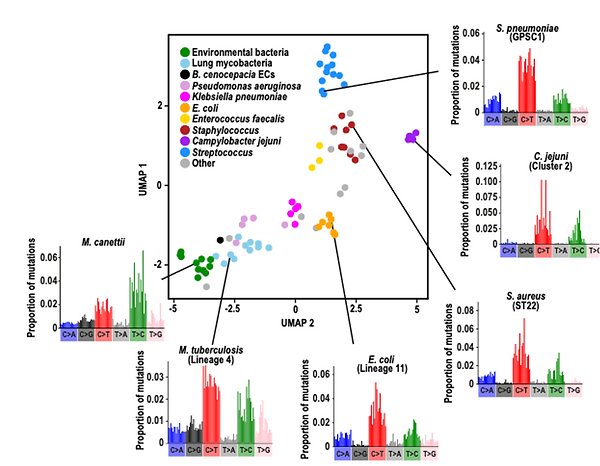
Ruis Christopher et al.,
Nature Communications 2023
"Mutational spectra are associated with bacterial niche"
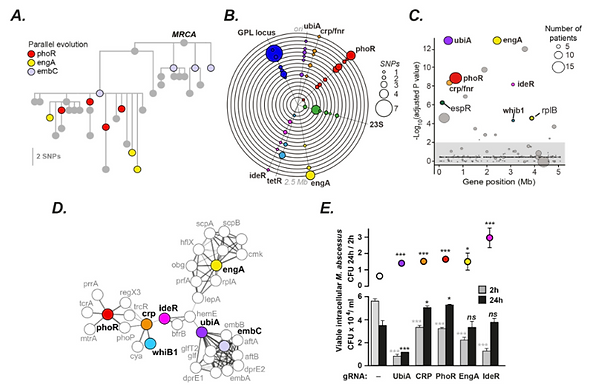
Bryant Josephine et al.,
Science 2021
"Stepwise pathogenic evolution of Mycobacterium abscessus"

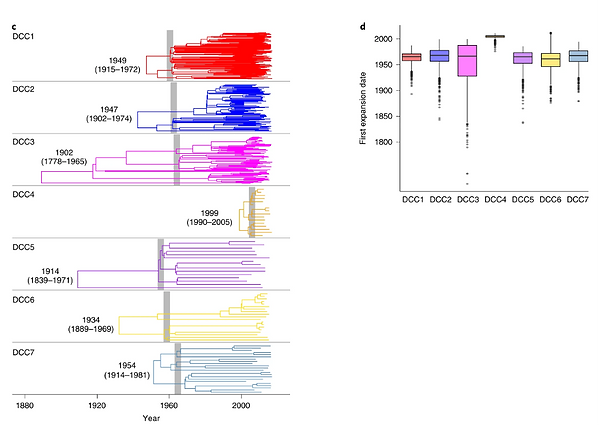
Boeck Lucas et al.,
Nature Microbiology 2022
"Mycobacterium abscessus pathogenesis identified by phenogenomic analyses"